In this second part of the series on structured concurrency (Part-I, Part-III, Part-IV, Swift-Followup), I will review Elixir and Erlang languages for writing concurrent applications and their support for structured concurrency:
Erlang
The Erlang language was created by late Joe Armstrong when he worked at Ericsson and it is designed for massive concurrency by means of very light weight processes that are based on actors. Each process has its own mailbox for storing incoming messages of various kinds. The receive block in Erlang is triggered upon new message arrival and the message is removed and executed when it matches specific pattern match. The Erlang language uses supervisors for monitoring processes and immutable functional paradigm for writing robust concurrent systems. Following is high-level architecture of Erlang system:
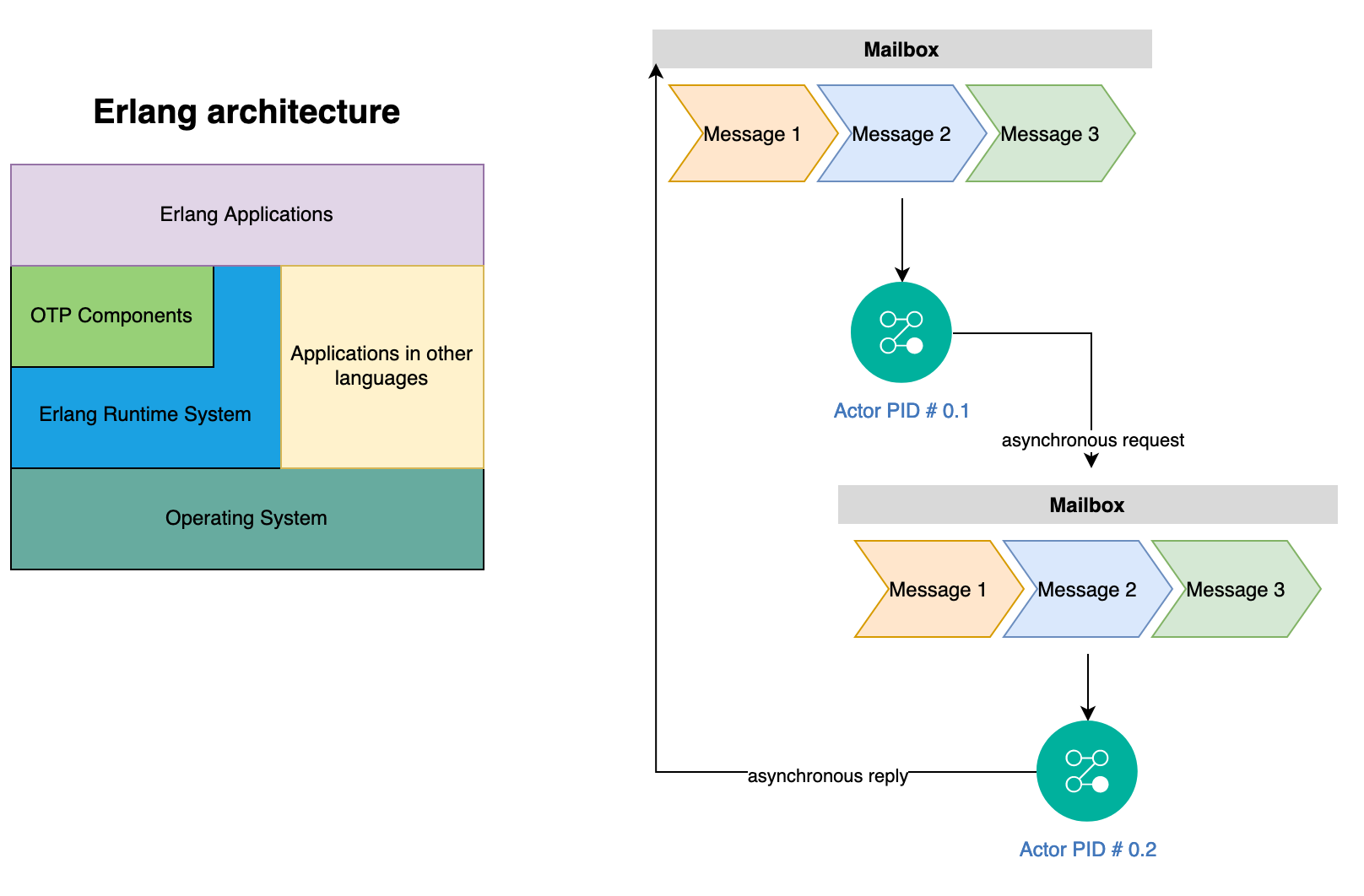
As the cost of each process or actor is only few hundred bytes, you can create millions of these processes for writing highly scalable concurrent systems. Erlang is a functional language where all data is immutable by default and the state within each actor is held private so there is no shared state or race conditions.
An actor keeps a mailbox for incoming messages and processes one message at a time using the receive API. Erlang doesn’t provide native async/await primitives but you can simulate async by sending an asynchronous message to an actor, which can then reply back to the sender using its process-id. The requester process can then block using receive API until reply is received. Erlang process model has better support for timeouts with receive API to exit early if it doesn’t receive response within a time period. Erlang system uses the mantra of let it crash for building fault tolerant applications and you can terminate a process and all children processes connected.
Using actor model in Erlang
Following code shows how native send and receive primitives can be used to build the toy web crawler:
-module(erlcrawler).
-export([start_link/0, crawl_urls/3, total_crawl_urls/1]).
-record(request, {clientPid, ref, url, depth, timeout, created_at=erlang:system_time(millisecond)}).
-record(result, {url, status=pending, child_urls=0, started_at=erlang:system_time(millisecond), completed_at, error}).
-define(MAX_DEPTH, 4).
-define(MAX_URL, 11).
-define(DOMAINS, [
"ab.com",
"bc.com",
"cd.com",
"de.com",
"ef.com",
"fg.com",
"yz.com"]).
make_request(ClientPid, Ref, Url, Depth, Timeout) ->
#request{clientPid=ClientPid, ref=Ref, url=Url, depth=Depth, timeout=Timeout}.
make_result(Req) ->
Url = Req#request.url,
#result{url=Url}.
%%% Client API
start_link() ->
spawn_link(fun init/0).
%%%%%%%%%%%% public method for crawling %%%%%%%%%%%%
%%% calling private method for crawling
%%% Pid - process-id of actor
%%% 0 - current depth
%%% Urls - list of urls to crawl
%%% Timeout - max timeout
crawl_urls(Pid, Urls, Timeout) when is_pid(Pid), is_list(Urls) ->
%% Boundary for concurrency and it will not return until all
%% child URLs are crawled up to MAX_DEPTH limit.
do_crawl_urls(Pid, 0, Urls, [], Timeout, 0).
total_crawl_urls(Pid) when is_pid(Pid) ->
Self = self(),
Pid ! {total, Self},
receive {total_reply, Self, N} ->
N
end.
%%% Server functions
init() ->
{ok, DownloaderPid} = downloader:start_link(),
{ok, IndexerPid} = indexer:start_link(),
loop(DownloaderPid, IndexerPid, 0).
%%% Main server loop
loop(DownloaderPid, IndexerPid, N) ->
receive
{crawl, Req} ->
CrawlerPid = self(),
spawn_link(fun() -> handle_crawl(CrawlerPid, Req, DownloaderPid, IndexerPid) end),
debug_print(N),
loop(DownloaderPid, IndexerPid, N+1);
{total, Pid} ->
Pid ! {total_reply, Pid, N},
loop(DownloaderPid, IndexerPid, N);
terminate ->
ok
end.
%%% Internal client functions
debug_print(N) when N rem 10000 == 0 ->
io:format("~p...~n", [{N}]);
debug_print(_) ->
ok.
%% Go through URLs to crawl, send asynchronous request to crawl and
%% then add request to a list to monitor that will be used to receive
%% reply back from the crawling actor.
do_crawl_urls(_, _, [], [], _, ChildURLs) ->
ChildURLs; % all done
do_crawl_urls(_, ?MAX_DEPTH, _, _, _, _) ->
0; % reached max depth, stop more crawling
do_crawl_urls(Pid, Depth, [Url|T], SubmittedRequests, Timeout, 0) when is_pid(Pid), is_integer(Depth), is_integer(Timeout) ->
%%% monitoring actor so that we are notified when actor process dies
Ref = erlang:monitor(process, Pid),
%%% crawling next url to process
Req = make_request(self(), Ref, Url, Depth, Timeout),
Pid ! {crawl, Req},
do_crawl_urls(Pid, Depth, T, SubmittedRequests ++ [Req], Timeout, 0);
do_crawl_urls(Pid, Depth, [], [Req|T], Timeout, ChildURLs) when is_pid(Pid) ->
%%% receiving response from the requests that were previously stored
Ref = Req#request.ref,
receive
{crawl_done, Ref, Res} ->
erlang:demonitor(Ref, [flush]),
do_crawl_urls(Pid, Depth, [], T, Timeout, Res#result.child_urls+ChildURLs+1);
{'DOWN', Ref, process, Pid, Reason} ->
erlang:error(Reason)
after Timeout ->
erlang:error({crawl_timeout, Timeout})
end.
%%% Internal server functions called by actor to process the crawling request
handle_crawl(CrawlerPid, Req, DownloaderPid, IndexerPid) ->
Res = make_result(Req),
ClientPid = Req#request.clientPid,
Url = Req#request.url,
Ref = Req#request.ref,
Depth = Req#request.depth,
Timeout = Req#request.timeout,
case downloader:download(DownloaderPid, Url) of
{ok, Contents} ->
{ok, Contents1} = downloader:jsrender(DownloaderPid, Url, Contents),
Changed = has_content_changed(Url, Contents1),
Spam = is_spam(Url, Contents1),
if Changed and not Spam ->
indexer:index(IndexerPid, Url, Contents1), % asynchronous call
Urls = parse_urls(Url, Contents1),
%% Crawling child urls synchronously before returning
ChildURLs = do_crawl_urls(CrawlerPid, Depth+1, Urls, [], Timeout, 0) + 1,
Res1 = Res#result{completed_at=erlang:system_time(millisecond), child_urls=ChildURLs},
ClientPid ! {crawl_done, Ref, Res1};
true ->
Res1 = Res#result{completed_at=erlang:system_time(millisecond)},
ClientPid ! {crawl_done, Ref, Res1}
end;
Err ->
Res1 = Res#result{completed_at=erlang:system_time(millisecond), error = Err},
ClientPid ! {crawl_done, Ref, Res1}
end,
ok.
%%%%%%%%%%%%%%% INTERNAL METHODS FOR CRAWLING %%%%%%%%%%%%%%%%
parse_urls(_Url, _Contents) ->
% tokenize contents and extract href/image/script urls
random_urls(?MAX_URL).
random_urls(N) ->
[random_url() || _ <- lists:seq(1, N)].
has_content_changed(_Url, _Contents) ->
% calculate hash digest and compare it with last digest
true.
is_spam(_Url, _Contents) ->
% apply standardize, stem, ngram, etc for indexing
false.
random_url() ->
"https://" ++ random_domain() ++ "/" ++ random_string(20).
random_domain() ->
lists:nth(random:uniform(length(?DOMAINS)), ?DOMAINS).
random_string(Length) ->
AllowedChars = "abcdefghijklmnopqrstuvwxyz",
lists:foldl(fun(_, Acc) -> [lists:nth(random:uniform(length(AllowedChars)), AllowedChars)] ++ Acc end, [], lists:seq(1, Length)).
In above implementation, crawl_urls method takes list of URLs and time out and waits until all URLs are crawled. It uses spawn_link to create a process, which invokes handle_crawl method to process requests concurrently. The handle_crawl method recursively crawl the URL and its children up to MAX_DEPTH limit. This implementation uses separate Erlang OTP processes for downloading, rendering and indexing contents. The handle_crawl sends back the response with number of child URLs that it crawled.
-module(erlcrawler_test).
-include_lib("eunit/include/eunit.hrl").
-define(ROOT_URLS, ["a.com", "b.com", "c.com", "d.com", "e.com", "f.com", "g.com", "h.com", "i.com", "j.com", "k.com", "l.com", "n.com"]).
crawl_urls_test() ->
{spawn, {timeout,30, do_crawl_urls(10000)}}.
%% Testing timeout and by default, it will terminate the test process so we will instead convert
%% kill signal into a message using erlang:exit
crawl_urls_with_timeout_test() ->
%%% crawling next url to process
Started = erlang:system_time(millisecond),
Timeout = 10, % We know that processing takes longer than 10 milliseconds
Pid = erlcrawler:start_link(),
process_flag(trap_exit, true),
spawn_link(fun() ->
erlcrawler:crawl_urls(Pid, ?ROOT_URLS, Timeout)
end),
{{crawl_timeout, _}, _} = receive
{'EXIT', _, Reason} -> Reason
after 1000 ->
erlang:error(unexpected_timeout)
end,
Elapsed = erlang:system_time(millisecond) - Started,
?debugFmt("crawl_urls_with_timeout_test: timedout as expected in millis ~p ~n", [{Elapsed}]).
%% Testing terminate/cancellation and killing a process will kill all its children
crawl_urls_with_terminate_test() ->
%%% crawling next url to process
Started = erlang:system_time(millisecond),
Pid = erlcrawler:start_link(),
spawn_link(fun() ->
erlcrawler:crawl_urls(Pid, ?ROOT_URLS, 1000) % crawl_urls is synchronous method so calling in another process
end),
receive
after 15 -> % waiting for a bit before terminating (canceling) process
exit(Pid, {test_terminated})
end,
{test_terminated} = receive
{'EXIT', Pid, Reason} -> Reason
after 200 ->
erlang:error(unexpected_timeout)
end,
Elapsed = erlang:system_time(millisecond) - Started,
?debugFmt("crawl_urls_with_terminate_test: terminated as expected in millis ~p ~n", [{Elapsed}]).
do_crawl_urls(Timeout) ->
Started = erlang:system_time(millisecond),
Pid = erlcrawler:start_link(),
N = erlcrawler:crawl_urls(Pid, ?ROOT_URLS, Timeout),
N1 = erlcrawler:total_crawl_urls(Pid),
Elapsed = erlang:system_time(millisecond) - Started,
?debugFmt("do_crawl_urls: Crawled URLs in millis: ~p ~n", [{N, N1, Elapsed}]),
?assertEqual(N1, 19032).
Above tests show three ways to try out the crawl_urls API. First test crawl_urls_test tests happy case of crawling URLs within 10 seconds. The crawl_urls_with_timeout_test tests the timeout behavior to make sure proper error message is returned and all Erlang processes are terminated. The crawl_urls_with_terminate_test tests cancellation behavior by terminating the main crawling process. You can download the full source code from https://github.com/bhatti/concurency-katas/tree/main/erl_actor.
Following are major benefits of using this process model to implement structured concurrency:
- The main crawl_urls method defines high level scope of concurrency and it waits for the completion of child tasks.
- crawl_urls method takes a timeout parameter so that the crawling all URLs must complete with the time period.
- Erlang allows parent-child relationship between processes where you can monitor child processes and get notified when a child process dies. You can use this feature to cancel the asynchronous task. However, it will abruptly end all processes and all state within the process will be lost.
- Erlang implementation captures the error within the response so the client can handle all error handling using pattern matching or other approach common in Erlang applications.
Following are shortcomings using this approach for structured concurrency:
- The terminate API is not suitable for clean cancellation so you will need to implement a cooperative cancellation to persist any state or clean up underlying resources.
- Though, you can combine processes in groups or parent child relationships manually but Erlang doesn’t give you a lot of flexibility to specify the context for execution.
- Unlike async declared methods in Typescript, Erlang code is not easily composable but you can define client code to wrap send/receive messages so that high level code can be comprehended easily. Also, Erlang processes can be connected with parent-child relationships and you can manage composition via process-supervisor hierarchy.
- Above code creates a new process for crawling each URL and though the overhead of each process is small but it may use other expensive resources such as network resource. We won’t use such approach for real crawler as it will strain the resources on the website being crawled. Instead, we may need to limit how many concurrent requests can be sent to a given website or maintain delay between successive requests.
Using pmap in Erlang
We can generalize above approach into a general purpose pmap that processes an array (similar to map function in functional languages) concurrently and then waits for their response such as:
-module(pmap).
-export([pmap/3]).
pmap(F, Es, Timeout) ->
Parent = self(),
Running = [exec(Parent, F, E) || E <- Es],
collect(Running, Timeout).
exec(Parent, F, E) ->
spawn_monitor(fun() -> Parent ! {self(), F(E)} end).
collect([], _Timeout) -> [];
collect([{Pid, MRef} | Next], Timeout) ->
receive
{Pid, Res} ->
erlang:demonitor(MRef, [flush]),
[{ok, Res} | collect(Next, Timeout)];
{'DOWN', MRef, process, Pid, Reason} ->
[{error, Reason} | collect(Next, Timeout)]
after Timeout ->
erlang:error({pmap_timeout, Timeout})
end.
You can download full pmap example from https://github.com/bhatti/concurency-katas/tree/main/erl_pmap.
Elixir
The Elixir language is built upon Erlang BEAM VM and was created by Jose Valim to improve usability of Erlang language and introduce Rubyist syntax instead of Prologist syntax in Erlang language. It also removes some of the boilerplate that you needed in Erlang language and adds higher level abstractions for writing highly concurrent, distributed and fault tolerant applications.
Using a worker-pool and OTP in Elixir
As Elixir uses Erlang VM and runtime system, the application behavior will be similar to Erlang applications but following approach uses a worker pool design where the parent process keeps a list of child-processes and delegates the crawling work to child processes in a round-robin fashion:
defmodule Crawler do
@max_depth 4
@moduledoc """
Documentation for Crawler.
"""
## Client API
# {:ok, pid} = Crawler.start_link(100000)
def start_link(size) when is_integer(size) do
GenServer.start_link(__MODULE__, size)
end
def total_crawl_urls(pid) when is_pid(pid) do
GenServer.call(pid, {:total_crawl_urls}, 30000)
end
### Public client APIs
def crawl_urls(pid, urls) when is_pid(pid) and is_list(urls) do
## Boundary for concurrency and it will not return until all
## child URLs are crawled up to MAX_DEPTH limit.
crawl_urls(pid, urls, 0, self())
end
### Internal client APIs
def crawl_urls(pid, urls, depth, clientPid) when is_pid(pid) and is_list(urls) do
if depth < @max_depth do
requests = urls |> Enum.map(&(Request.new(&1, depth, clientPid)))
requests |> Enum.map(&(GenServer.cast(pid, {:crawl, &1})))
else
:max_depth_exceeded
end
end
## init method create pool of workers based on given size
def init(size) when is_integer(size) do
Process.flag(:trap_exit, true)
pid_to_workers = 0..size |> Enum.map(&child_spec/1)
|> Enum.map(&start_child/1)
|> Enum.into(%{})
pids = Map.keys(pid_to_workers)
{:ok, {pid_to_workers, pids, 0}}
end
## handles crawling
def handle_cast({:crawl, request}, {pid_to_workers, [pid|rest], total_in}) do
GenServer.cast(pid, {:crawl, request}) # send request to workers in round-robin fashion
{:noreply, {pid_to_workers, rest ++ [pid], total_in+1}}
end
def handle_call({:total_crawl_urls}, _from, {_, _, total_in} = state) do
{:reply, total_in, state}
end
## OTP Callbacks
def handle_info({:EXIT, dead_pid, _reason}, {pid_to_workers, _, total_in}) do
# Start new process based on dead_pid spec
{new_pid, child_spec} = pid_to_workers
|> Map.get(dead_pid)
|> start_child()
# Remove the dead_pid and insert the new_pid with its spec
new_pid_to_workers = pid_to_workers
|> Map.delete(dead_pid)
|> Map.put(new_pid, child_spec)
pids = Map.keys(new_pid_to_workers)
{:noreply, {new_pid_to_workers, pids, total_in}}
end
## Defines spec for worker
defp child_spec(_) do
{Worker, :start_link, [self()]}
end
## Dynamically create child
defp start_child({module, function, args} = spec) do
{:ok, pid} = apply(module, function, args)
Process.link(pid)
{pid, spec}
end
end
The parent process in above example defines crawl_urls method for crawling URLs, which is defined as an asynchronous API (handle_cast) and forwards the request to next worker. Following is implementation of the worker:
defmodule Worker do
@moduledoc """
Documentation for crawling worker.
"""
@max_url 11
@domains [
"ab.com",
"bc.com",
"cd.com",
"de.com",
"yz.com"]
@allowed_chars "abcdefghijklmnopqrstuvwxyz"
use GenServer
# Client APIs
def start_link(crawler_pid) when is_pid(crawler_pid) do
{:ok, downloader_pid} = Downloader.start_link()
{:ok, indexer_pid} = Indexer.start_link()
GenServer.start_link(__MODULE__, {crawler_pid, downloader_pid, indexer_pid})
end
@doc """
Crawls web url asynchronously
"""
def handle_cast({:crawl, request}, {crawler_pid, downloader_pid, indexer_pid}=state) do
handle_crawl(crawler_pid, downloader_pid, indexer_pid, request)
{:noreply, state}
end
def init(crawler_pid) do
{:ok, crawler_pid}
end
# Internal private methods
defp handle_crawl(crawler_pid, downloader_pid, indexer_pid, req) do
res = Result.new(req)
contents = Downloader.download(downloader_pid, req.url)
new_contents = Downloader.jsrender(downloader_pid, req.url, contents)
if has_content_changed(req.url, new_contents) and !is_spam(req.url, new_contents) do
Indexer.index(indexer_pid, req.url, new_contents)
urls = parse_urls(req.url, new_contents)
Crawler.crawl_urls(crawler_pid, urls, req.depth+1, req.clientPid)
send req.clientPid, {:crawl_done, Result.completed(res)}
else
send req.clientPid, {:crawl_done, Result.failed(req, :skipped_crawl)}
end
end
defp parse_urls(_Url, _Contents) do
# tokenize contents and extract href/image/script urls
random_urls(@max_url)
end
defp random_urls(n) do
1..n |> Enum.map(&(random_url/1))
end
defp has_content_changed(_url, _contents) do
# calculate hash digest and compare it with last digest
true
end
defp is_spam(_url, _contents) do
# apply standardize, stem, ngram, etc for indexing
false
end
defp random_url(_) do
"https://" <> random_domain() <> "/" <> random_string(20)
end
defp random_domain() do
Enum.random(@domains)
end
defp random_string(n) do
1..n
|> Enum.reduce([], fn(_, acc) -> [Enum.random(to_charlist(@allowed_chars)) | acc] end)
|> Enum.join("")
end
end
The worker process starts downloader and indexer processes upon start and crawls the URL upon receiving the next request. It then sends back the response to the originator of request using process-id in the request. Following unit tests are used to test the behavior of normal processing, timeouts and cancellation:
defmodule CrawlerTest do
use ExUnit.Case
doctest Crawler
@max_processes 10000
@max_wait_messages 19032
@root_urls ["a.com", "b.com", "c.com", "d.com", "e.com", "f.com", "g.com", "h.com", "i.com", "j.com", "k.com", "l.com", "n.com"]
test "test crawling urls" do
started = System.system_time(:millisecond)
{:ok, pid} = Crawler.start_link(@max_processes)
Crawler.crawl_urls(pid, @root_urls)
wait_until_total_crawl_urls(pid, @max_wait_messages, started)
end
defp wait_until_total_crawl_urls(pid, 0, started) do
n = Crawler.total_crawl_urls(pid)
elapsed = System.system_time(:millisecond) - started
IO.puts("Crawled URLs in millis: #{n} #{elapsed}")
assert n >= @max_wait_messages
end
defp wait_until_total_crawl_urls(pid, max, started) do
if rem(max, 1000) == 0 do
IO.puts("#{max}...")
end
receive do
{:crawl_done, _} -> wait_until_total_crawl_urls(pid, max-1, started)
end
end
end
Following are major benefits of this approach for its support of structured concurrency:
- The crawl_urls method in parent process defines high level scope of concurrency and it waits for the completion of child tasks.
- Above implementation also uses timeout similar to the Erlang example to ensure task is completed within given time period.
- Above implementation also captures the error within the response similar to Erlang for error handling.
- This approach addresses some of the shortcomings of previous approach in Erlang implementation where a new process was created for each request. Instead a pool of process is used to manage the capacity of resources.
Following are shortcomings using this approach for structured concurrency:
- This approach also suffers the same drawbacks as Erlang approach regarding cancellation behavior and you will need to implement a cooperative cancellation to cleanup the resources properly.
- Similar to Erlang, Elixir also doesn’t give you a lot of flexibility to specify the context for execution and it’s not easily composable.
Using async-await in Elixir
Elixir defines abstracts Erlang process with Task when you only need to execute a single action throughout its lifetime. Here is an example that combines Task async/await with pmap implementation:
defmodule Parallel do
def pmap(collection, func, timeout) do
collection
|> Enum.map(&(Task.async(fn -> func.(&1) end)))
|> Enum.map(fn t -> Task.await(t, timeout) end)
end
end
defmodule Crawler do
@domains [
"ab.com",
"bc.com",
"cd.com",
"de.com",
"ef.com",
"yz.com"]
@allowed_chars "abcdefghijklmnopqrstuvwxyz"
@max_depth 4
@max_url 11
@moduledoc """
Documentation for Crawler.
"""
## Client API
def crawl_urls(urls, timeout) when is_list(urls) do
## Boundary for concurrency and it will not return until all
## child URLs are crawled up to MAX_DEPTH limit.
## Starting external services using OTP for downloading and indexing
{:ok, downloader_pid} = Downloader.start_link()
{:ok, indexer_pid} = Indexer.start_link()
res = crawl_urls(urls, downloader_pid, indexer_pid, 0, timeout)
## Stopping external services using OTP for downloading and indexing
Process.exit(downloader_pid, :normal)
Process.exit(indexer_pid, :normal)
res
end
def crawl_urls(urls, downloader_pid, indexer_pid, depth, timeout) when is_list(urls) and is_pid(downloader_pid) and is_pid(indexer_pid) and is_integer(depth) and is_integer(timeout) do
if depth < @max_depth do
requests = urls |> Enum.map(&(Request.new(&1, downloader_pid, indexer_pid, depth, timeout)))
Parallel.pmap(requests, &(handle_crawl/1), timeout)
else
[]
end
end
# Internal private methods
defp handle_crawl(req) do
{:ok, contents} = Downloader.download(req.downloader_pid, req.url, req.timeout)
{:ok, new_contents} = Downloader.jsrender(req.downloader_pid, req.url, contents, req.timeout)
if has_content_changed(req.url, new_contents) and !is_spam(req.url, new_contents) do
Indexer.index(req.indexer_pid, req.url, new_contents, req.timeout)
urls = parse_urls(req.url, new_contents)
res = Crawler.crawl_urls(urls, req.downloader_pid, req.indexer_pid, req.depth+1, req.timeout)
Enum.reduce(res, 0, &(&1 + &2)) + 1
else
0
end
end
defp parse_urls(_Url, _Contents) do
# tokenize contents and extract href/image/script urls
random_urls(@max_url)
end
defp random_urls(n) do
1..n |> Enum.map(&(random_url/1))
end
defp has_content_changed(_url, _contents) do
# calculate hash digest and compare it with last digest
true
end
defp is_spam(_url, _contents) do
# apply standardize, stem, ngram, etc for indexing
false
end
defp random_url(_) do
"https://" <> random_domain() <> "/" <> random_string(20)
end
defp random_domain() do
Enum.random(@domains)
end
defp random_string(n) do
1..n
|> Enum.reduce([], fn(_, acc) -> [Enum.random(to_charlist(@allowed_chars)) | acc] end)
|> Enum.join("")
end
end
Above example is a bit shorter due to the high level Task abstraction but its design has similar pros/cons as actor and pmap implementation of Erlang example. You can download full source code for this implementation from https://github.com/bhatti/concurency-katas/tree/main/elx_pmap.
Using Queue in Elixir
Following example shows web crawler implementation using queue:
defmodule Crawler do
@max_depth 4
@moduledoc """
Documentation for Crawler.
"""
## Client API
def start_link(size) when is_integer(size) do
{:ok, downloader_pid} = Downloader.start_link()
{:ok, indexer_pid} = Indexer.start_link()
GenServer.start_link(__MODULE__, {size, downloader_pid, indexer_pid})
end
## crawl list of url
def crawl_urls(pid, urls, timeout) when is_pid(pid) and is_list(urls) and is_integer(timeout) do
## Boundary for concurrency and it will not return until all
## child URLs are crawled up to MAX_DEPTH limit.
crawl_urls(pid, urls, 0, self(), timeout)
end
# returns number of urls crawled
def total_crawl_urls(pid, timeout) when is_pid(pid) do
GenServer.call(pid, {:total_crawl_urls}, timeout)
end
## dequeue returns pops top request from the queue and returns it
def dequeue(pid) when is_pid(pid) do
GenServer.call(pid, {:dequeue})
end
###########################################
## internal api to crawl urls
def crawl_urls(pid, urls, depth, clientPid, timeout) when is_pid(pid) and is_list(urls) and is_pid(clientPid) and is_integer(timeout) do
if depth < @max_depth do
requests = urls |> Enum.map(&(Request.new(&1, depth, clientPid, timeout)))
requests |> Enum.map(&(GenServer.cast(pid, {:crawl, &1})))
else
:max_depth_exceeded
end
end
###########################################
## init method create pool of workers based on given size
def init({size, downloader_pid, indexer_pid}) when is_integer(size) and is_pid(downloader_pid) and is_pid(indexer_pid) do
Process.flag(:trap_exit, true)
pid_to_workers = 0..size |> Enum.map(&child_spec/1)
|> Enum.map(&start_child/1)
|> Enum.into(%{})
{:ok, {pid_to_workers, :queue.new, 0, 0, downloader_pid, indexer_pid}}
end
## asynchronous server handler for adding request to crawl in the queue
def handle_cast({:crawl, request}, {pid_to_workers, queue, total_in, total_out, downloader_pid, indexer_pid}) do
new_queue = :queue.in(request, queue)
{:noreply, {pid_to_workers, new_queue, total_in+1, total_out, downloader_pid, indexer_pid}}
end
## synchronous server handler for returning total urls crawled
def handle_call({:total_crawl_urls}, _from, {_, _, _total_in, total_out, _, _} = state) do
{:reply, total_out, state}
end
## synchronous server handler to pop top request from the queue and returning it
def handle_call({:dequeue}, _from, {pid_to_workers, queue, total_in, total_out, downloader_pid, indexer_pid}) do
{head, new_queue} = :queue.out(queue)
if head == :empty do
{:reply, {head, downloader_pid, indexer_pid}, {pid_to_workers, new_queue, total_in, total_out, downloader_pid, indexer_pid}}
else
if rem(:queue.len(queue), 1000) == 0 or rem(total_out+1, 1000) == 0do
IO.puts("#{total_out+1}...")
end
{:value, req} = head
{:reply, {req, downloader_pid, indexer_pid}, {pid_to_workers, new_queue, total_in, total_out+1, downloader_pid, indexer_pid}}
end
end
## OTP helper callbacks
def handle_info({:EXIT, dead_pid, _reason}, {pid_to_workers, queue, total_in, total_out}) do
# Start new process based on dead_pid spec
{new_pid, child_spec} = pid_to_workers
|> Map.get(dead_pid)
|> start_child()
# Remove the dead_pid and insert the new_pid with its spec
new_pid_to_workers = pid_to_workers
|> Map.delete(dead_pid)
|> Map.put(new_pid, child_spec)
{:noreply, {new_pid_to_workers, queue, total_in, total_out}}
end
## Defines spec for worker
defp child_spec(_) do
{Worker, :start_link, [self()]}
end
## Dynamically create child
defp start_child({module, function, args} = spec) do
{:ok, pid} = apply(module, function, args)
Process.link(pid)
{pid, spec}
end
end
You can download full source code of this example from https://github.com/bhatti/concurency-katas/tree/main/elx_queue.
Using Actor model as Abstract Data Structure
As the cost of actors is very small, you can also use it as an abstract data structure or objects that maintains internal state. Alan Kay, the pioneer in object-oriented programming described message-passing, isolation and state encapsulation as foundation of object-oriented design and Joe Armstrong described Erlang as the only object-oriented language. For example, let’s say you need to create a cache of stock quotes using dictionary data structure, which is updated from another source and provides easy access to the latest quotes. You would need to protect access to shared data in multi-threaded environment with synchronization. However, with actor-based design, you may define an actor for each stock symbol that keeps latest value internally and provides API to access or update quote data. This design will remove the need to synchronize shared data structure and will result in better performance.
Overall, Erlang process model is a bit low-level compared to async/await syntax and lacks composition in asynchronous code but it can be designed to provide structured scope, error handling and termination. Further, immutable data structures and message passing obviates the need for locks to protect shared state. Another benefit of Erlang/Elixir is its support of distributed services so it can be used for automatically distributing tasks to remote machines seamlessly.