Though, REST standard for remote APIs is fairly loose but you can document API shape and structure using standards such as Open API and swagger specifications. The documented API specification ensures that both consumer/client and producer/server side abide by the specifications and prevent unexpected behavior. The API provider may also define service-level objective (SLO) so that API meets specified latency, security and availability and other service-level indicators (SLI). The API provider can use contract tests to validate the API interactions based on documented specifications. The contract testing includes both consumer and producer where a consumer makes an API request and the producer produces the result. The contract tests ensures that both consumer requests and producer responses match the contract request and response definitions per API specifications. These contract tests don’t just validate API schema instead they validate interactions between consumer and producer thus they can also be used to detect any breaking or backward incompatible changes so that consumers can continue using the APIs without any surprises.
In order to demonstrate contract testing, we will use api-mock-service library to generate mock/stub client requests and server responses based on Open API specifications or customized test contracts. These test contracts can be used by both consumers and producers for validating API contracts and evolve the contract tests as API specifications are updated.
Sample REST API Under Test
A sample eCommerce application will be used to demonstrate contracts testing. The application will use various REST APIs to implement online shopping experience. The primary purpose of this example is to show how different request structures can be passed to the REST APIs and then generate a valid result or an error condition for contract testing. You can view the Open-API specifications for this sample app here.
Customer REST APIs
The customer APIs define operations to manage customers who shop online, e.g.:
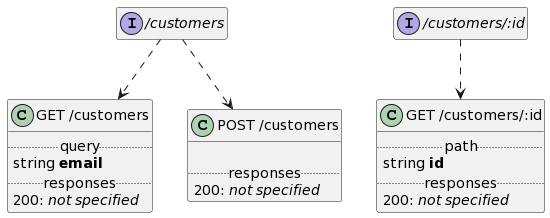
Product REST APIs
The product APIs define operations to manage products that can be shopped online, e.g.:

Payment REST APIs
The payment APIs define operations to charge credit card and pay for online shopping, e.g.:
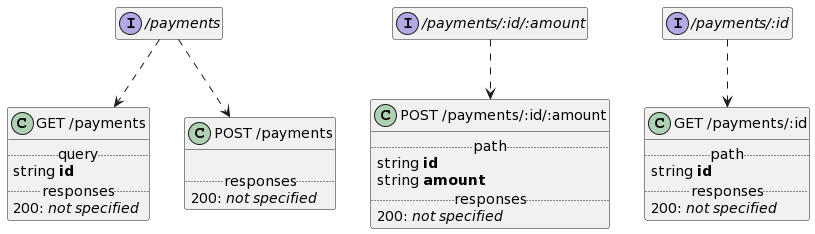
Order REST APIs
The order APIs define operations to purchase a product from the online store and it will use above APIs to validate customers, check product inventory, charge payments and then store record of orders, e.g.:

Generating Stub Server Responses based on Open-API Specifications
In this example, stub server responses will be generated by api-mock-service based on open-api specifications ecommerce-api.json by starting the mock service first as follows:
docker pull plexobject/api-mock-service:latest
docker run -p 8000:8000 -p 9000:9000 -e HTTP_PORT=8000 -e PROXY_PORT=9000 \
-e DATA_DIR=/tmp/mocks -e ASSET_DIR=/tmp/assets api-mock-service
And then uploading open-API specifications for ecommerce-api.json:
curl -H "Content-Type: application/yaml" --data-binary @ecommerce-api.json \
http://localhost:8000/_oapi
It will generate test contracts with stub/mock responses for all APIs defined in the ecommerce-api.json Open API specification. For example, you can produce result of customers REST APIs, e.g.:
curl http://localhost:8000/customers
to produce:
[
{
"address": {
"city": "PpCJyfKUomUOdhtxr",
"countryCode": "US",
"id": "ede97f59-2ef2-48e5-913f-4bce0f152603",
"streetAddress": "Se somnis cibo oculi, die flammam petimus?",
"zipCode": "06826"
},
"creditCard": {
"balance": {
"amount": 53965,
"currency": "CAD"
},
"cardNumber": "7345-4444-5461",
"customerId": "WB97W4L2VQRRkH5L0OAZGk0MT957r7Z",
"expiration": "25/0000",
"id": "ae906a78-0aff-4d4e-ad80-b77877f0226c",
"type": "VISA"
},
"email": "abigail.appetitum@dicant.net",
"firstName": "sciam",
"id": "21c82838-507a-4745-bc1b-40e6e476a1fb",
"lastName": "inquit",
"phone": "1-717-5555-3010"
},
Above response is randomly generated based on the types/formats/regex/min-max limits of properties defined in Open-API and calling this API will automatically generate all valid and error responses, e.g. calling “curl http://localhost:8000/
customers” again will return:
* Mark bundle as not supporting multiuse
< HTTP/1.1 500 Internal Server Error
< Content-Type:
< Vary: Origin
< X-Mock-Path: /customers
< X-Mock-Request-Count: 9
< X-Mock-Scenario: getCustomerByEmail-customers-500-8a93b6c60c492e730ea149d5d09e79d85701c01dbc017d178557ed1d2c1bad3d
< Date: Sun, 01 Jan 2023 20:41:17 GMT
< Content-Length: 67
<
* Connection #0 to host localhost left intact
{"logRef":"achieve_output_fresh","message":"buffalo_rescue_street"}
Consumer-driven Contract Testing
Upon uploading the Open-API specifications of microservices, the api-mock-service generates test contracts for each REST API and response statuses. You can then customize these test cases for consumer-driven contract testing.

For example, here is the default test contract generated for finding a customer by id with path “/customers/:id”:
method GET
name getCustomer-customers-200-61a298e
path /customers/ id
description""
predicate""
request
match_query_params
match_headers
match_contents'{}'
path_params
id \w+
query_params
headers
response
headers
Content-Type
application/json
contents'{"address":{"city":"{{RandStringMinMax 2 60}}","countryCode":"{{EnumString `US CA`}}","id":"{{UUID}}","streetAddress":"{{RandRegex `\\w+`}}","zipCode":"{{RandRegex `\\d{5}`}}"},"creditCard":{"balance":{"amount":{{RandNumMinMax 0 0}},"currency":"{{RandRegex `(USD|CAD|EUR|AUD)`}}"},"cardNumber":"{{RandRegex `\\d{4}-\\d{4}-\\d{4}`}}","customerId":"{{RandStringMinMax 30 36}}","expiration":"{{RandRegex `\\d{2}/\\d{4}`}}","id":"{{UUID}}","type":"{{EnumString `VISA MASTERCARD AMEX`}}"},"email":"{{RandRegex `.+@.+\\..+`}}","firstName":"{{RandRegex `\\w`}}","id":"{{UUID}}","lastName":"{{RandRegex `\\w`}}","phone":"{{RandRegex `1-\\d{3}-\\d{4}-\\d{4}`}}"}'
contents_file""
status_code200
wait_before_reply 0s
Above template demonstrates interaction between consumer and producer by defining properties such as:
- method – of REST API such as GET/POST/PUT/DELETE
- name – of the test case
- path of REST API
- description – of test
- predicate – defines a condition which must be true to select this test contract
- request section defines input properties for the REST API including:
- match_query_params – to match query input parameters for selecting the test contract
- match_headers – to match input headers for selecting the test contract
- match_contents – defines regex for selecting input body
- path_params – defines path variables and regex
- query_params and headers – defines sample input parameters and headers
- response section defines output properties for the REST API including:
- headers – defines response headers
- contents – defines body of response
- contents_file – allows loading response from a file
- status_code – defines HTTP response status
- wait_before_reply – defines wait time before returning response
You can then invoke test contract using:
curl http://localhost:8000/customers/1
that generates test case from the mock/stub server provided by the api-mock-service library, e.g.
{
"address": {
"city": "PanHQyfbHZVw",
"countryCode": "US",
"id": "ff5d0e98-daa5-49c8-bb79-f2d7274f2fb1",
"streetAddress": "Sumus o proferens etiamne intuerer fugasti, nuntiantibus da?",
"zipCode": "01364"
},
"creditCard": {
"balance": {
"amount": 80704,
"currency": "USD"
},
"cardNumber": "3226-6666-2214",
"customerId": "0VNf07XNWkLiIBhfmfCnrE1weTlkhmxn",
"expiration": "24/5555",
"id": "f9549ef3-a5eb-4df4-a8a9-85a30a6a49c6",
"type": "VISA"
},
"email": "amanda.doleat@fructu.com",
"firstName": "quaero",
"id": "9aeee733-932d-4244-a6f8-f21d2883fd27",
"lastName": "habeat",
"phone": "1-052-5555-4733"
}
You can customize above response contents using builtin template functions in the api-mock-service library or create additional test contracts for each distinct input parameter. For example, following contract defines interaction between consumer and producer to add a new customer:
method POST
name saveCustomer-customers-200-ddfceb2
path /customers
description""
order0
group Sample Ecommerce API
predicate""
request
match_query_params
match_headers
match_contents'{"address.city":"(__string__\\w+)","address.countryCode":"(__string__(US|CA))","address.streetAddress":"(__string__\\w+)","address.zipCode":"(__string__\\d{5})","creditCard.balance.amount":"(__number__[+-]?((\\d{1,10}(\\.\\d{1,5})?)|(\\.\\d{1,10})))","creditCard.balance.currency":"(__string__(USD|CAD|EUR|AUD))","creditCard.cardNumber":"(__string__\\d{4}-\\d{4}-\\d{4})","creditCard.customerId":"(__string__\\w+)","creditCard.expiration":"(__string__\\d{2}/\\d{4})","creditCard.type":"(__string__(VISA|MASTERCARD|AMEX))","email":"(__string__.+@.+\\..+)","firstName":"(__string__\\w)","lastName":"(__string__\\w)","phone":"(__string__1-\\d{3}-\\d{4}-\\d{4})"}'
path_params
query_params
headers
ContentsType application/json
contents'{"address":{"city":"__string__\\w+","countryCode":"__string__(US|CA)","streetAddress":"__string__\\w+","zipCode":"__string__\\d{5}"},"creditCard":{"balance":{"amount":"__number__[+-]?((\\d{1,10}(\\.\\d{1,5})?)|(\\.\\d{1,10}))","currency":"__string__(USD|CAD|EUR|AUD)"},"cardNumber":"__string__\\d{4}-\\d{4}-\\d{4}","customerId":"__string__\\w+","expiration":"__string__\\d{2}/\\d{4}","type":"__string__(VISA|MASTERCARD|AMEX)"},"email":"__string__.+@.+\\..+","firstName":"__string__\\w","lastName":"__string__\\w","phone":"__string__1-\\d{3}-\\d{4}-\\d{4}"}'
example_contents
address:
city: Ab fabrorum meminerim conterritus nota falsissime deum?
countryCode: CA
streetAddress: Mei nisi dum, ab amaremus antris?
zipCode: "00128"
creditCard:
balance:
amount: 3000.4861560368768
currency: USD
cardNumber: 7740-7777-6114
customerId: Fudi eodem sed habitaret agam pro si?
expiration: 85/2222
type: AMEX
email: larry.neglecta@audio.edu
firstName: fatemur
lastName: gaudeant
phone: 1-543-8888-2641
response
headers
Content-Type
application/json
contents'{"address":{"city":"{{RandStringMinMax 2 60}}","countryCode":"{{EnumString `US CA`}}","id":"{{UUID}}","streetAddress":"{{RandRegex `\\w+`}}","zipCode":"{{RandRegex `\\d{5}`}}"},"creditCard":{"balance":{"amount":{{RandNumMinMax 0 0}},"currency":"{{RandRegex `(USD|CAD|EUR|AUD)`}}"},"cardNumber":"{{RandRegex `\\d{4}-\\d{4}-\\d{4}`}}","customerId":"{{RandStringMinMax 30 36}}","expiration":"{{RandRegex `\\d{2}/\\d{4}`}}","id":"{{UUID}}","type":"{{EnumString `VISA MASTERCARD AMEX`}}"},"email":"{{RandRegex `.+@.+\\..+`}}","firstName":"{{RandRegex `\\w`}}","id":"{{UUID}}","lastName":"{{RandRegex `\\w`}}","phone":"{{RandRegex `1-\\d{3}-\\d{4}-\\d{4}`}}"}'
contents_file""
status_code200
wait_before_reply 0s
Above template defines interaction for adding a new customer where request section defines format of request and matching criteria using match_content property. The response section includes the headers and contents that are generated by the stub/mock server for consumer-driven contract testing. You can then invoke test contract using:
curl -X POST http://localhost:8000/customers -d '{"address":{"city":"rwjJS","countryCode":"US","id":"4a788c96-e532-4a97-9b8b-bcb298636bc1","streetAddress":"Cura diu me, miserere me?","zipCode":"24121"},"creditCard":{"balance":{"amount":57012,"currency":"USD"},"cardNumber":"5566-2222-8282","customerId":"tgzwgThaiZqc5eDwbKk23nwjZqkap7","expiration":"70/6666","id":"d966aafa-c28b-4078-9e87-f7e9d76dd848","type":"VISA"},"email":"andrew.recorder@ipsas.net","firstName":"quendam","id":"071396bb-f8db-489d-a8f7-bbcce952ecef","lastName":"formaeque","phone":"1-345-6666-0618"}'
Which will return a response such as:
{
"address": {
"city": "j77oUSSoB5lJCUtc4scxtm0vhilPRdLE7Nc8KzAunBa87OrMerCZI",
"countryCode": "CA",
"id": "9bb21030-29d0-44be-8f5a-25855e38c164",
"streetAddress": "Qui superbam imago cernimus, sensarum nuntii tot da?",
"zipCode": "08020"
},
"creditCard": {
"balance": {
"amount": 75666,
"currency": "AUD"
},
"cardNumber": "1383-8888-5013",
"customerId": "nNaUd15lf6lqkAEwKoguVTvBnPMBVDhdeO",
"expiration": "73/5555",
"id": "554efad7-17ab-49f9-967a-3e47381a4d34",
"type": "AMEX"
},
"email": "deborah.vivit@desivero.gov",
"firstName": "contexo",
"id": "db70b737-ee1d-48ed-83da-c5a8773c7a5f",
"lastName": "delectat",
"phone": "1-013-7777-0054"
}
Note: The response will not match the request body as the contract testing only tests interactions between consumer and producer without maintaining any server side state. You can use other types of testing such as integration/component/functional testing for validating state based behavior.
Producer-driven Generated Tests
The process of defining contracts to generate tests for validating producer REST APIs is similar to consumer-driven contracts. For example, you can upload open-api specifications or user-defined contracts to the api-mock-service provided mock/stub server.
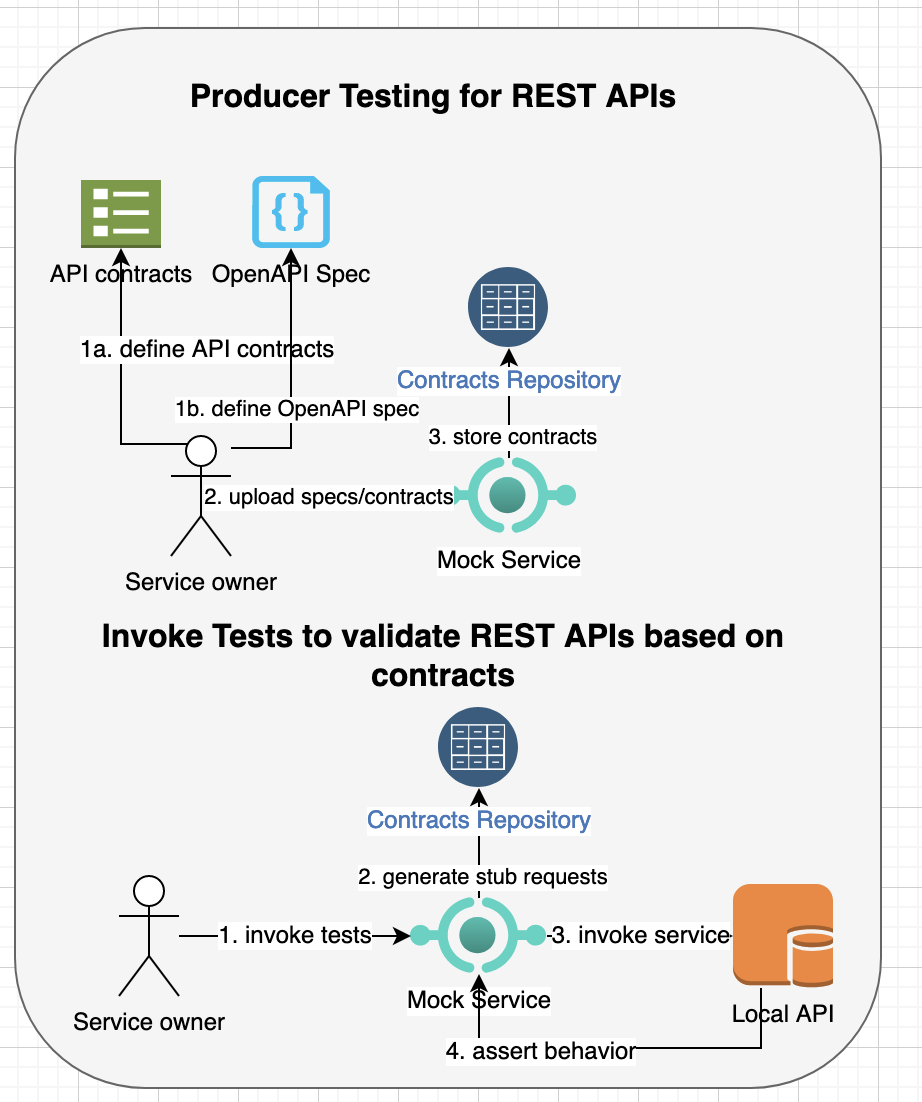
For example, you can upload open-API specifications for ecommerce-api.json as follows:
curl -H "Content-Type: application/yaml" --data-binary @ecommerce-api.json \
http://localhost:8000/_oapi
Upon uploading the specifications, the mock server will generate contracts for each REST API and status. You can customize those contracts with additional validation or assertion and then invoke server generated tests either by specifying the REST API or invoke multiple REST APIs belonging to a specific group. You can also define an order for executing tests in a group and can optionally pass data from one invocation to the next invocation of REST API.
For testing purpose, we will customize customer REST APIs for adding a new customer and fetching a customer by its id, i.e.,
A contract for adding a new customer
method POST
name save-customer
path /customers
group customers
order0
request
headers
Content-Type application/json
contents
address:
city: {{RandCity}}
countryCode: {{EnumString `US CA`}}
id: {{UUID}}
streetAddress: {{RandSentence 2 3}}
zipCode: {{RandRegex `\d{5}`}}
creditCard:
balance:
amount: {{RandNumMinMax 20 500}}
currency: {{EnumString `USD CAD`}}
cardNumber: {{RandRegex `\d{4}-\d{4}-\d{4}`}}
customerId: {{UUID}}
expiration: {{RandRegex `\d{2}/\d{4}`}}
id: {{UUID}}
type: {{EnumString `VISA MASTERCARD`}}
email: {{RandEmail}}
firstName: {{RandName}}
id: {{UUID}}
lastName: {{RandName}}
phone: {{RandRegex `1-\d{3}-\d{3}-\d{4}`}}
response
match_headers
match_contents'{"address.city":"(__string__\\w+)","address.countryCode":"(__string__(US|CA))","address.id":"(__string__\\w+)","address.streetAddress":"(__string__\\w+)","address.zipCode":"(__string__\\d{5}.?\\d{0,4})","creditCard.balance.amount":"(__number__[+-]?(([0-9]{1,10}(\\.[0-9]{1,5})?)|(\\.[0-9]{1,10})))","creditCard.balance.currency":"(__string__\\w+)","creditCard.cardNumber":"(__string__[\\d-]{10,20})","creditCard.customerId":"(__string__\\w+)","creditCard.expiration":"(__string__\\d{2}.\\d{4})","creditCard.id":"(__string__\\w+)","creditCard.type":"(__string__(VISA|MASTERCARD|AMEX))","email":"(__string__.+@.+\\..+)","firstName":"(__string__\\w+)","id":"(__string__\\w+)","lastName":"(__string__\\w+)","phone":"(__string__[\\-\\w\\d]{9,15})"}'
pipe_properties
id
email
assertions
VariableContains contents.email @
VariableContains contents.creditCard.type A
VariableContains headers.Content-Type application/json
VariableEQ status 200
The request section defines content property that will build the input request, which will be sent to the producer provided REST API. The server section defines match_contents to match regex of each response property. In addition, the response section defines assertions to compare against response contents, headers or status against expected output.
A contract for finding an existing customer
method GET
name get-customer
path /customers/ .id
description""
order1
group customers
predicate""
request
path_params
id \w+
query_params
headers
Content-Type application/json
contents""
example_contents""
response
headers
match_headers
Content-Type application/json
match_contents'{"address.city":"(__string__\\w+)","address.countryCode":"(__string__(US|CA))","address.streetAddress":"(__string__\\w+)","address.zipCode":"(__string__\\d{5})","creditCard.balance.amount":"(__number__[+-]?((\\d{1,10}(\\.\\d{1,5})?)|(\\.\\d{1,10})))","creditCard.balance.currency":"(__string__(USD|CAD|EUR|AUD))","creditCard.cardNumber":"(__string__\\d{4}-\\d{4}-\\d{4})","creditCard.customerId":"(__string__\\w+)","creditCard.expiration":"(__string__\\d{2}/\\d{4})","creditCard.type":"(__string__(VISA|MASTERCARD|AMEX))","email":"(__string__.+@.+\\..+)","firstName":"(__string__\\w)","lastName":"(__string__\\w)","phone":"(__string__1-\\d{3}-\\d{3}-\\d{4})"}'
pipe_properties
id
email
assertions
VariableContains contents.email @
VariableContains contents.creditCard.type A
VariableContains headers.Content-Type application/json
VariableEQ status 200
Above template defines similar properties to generate request body and defines match_contents with assertions to match expected output headers, body and status. Based on order of tests, the generated test to add new customer will be executed first, which will be followed by the test to find a customer by id. As we are testing against real REST APIs, the REST API path is defined as “/customers/{{.id}}” for finding a customer will populate the id from the output of first test based on the pipe_properties.
Uploading Contracts
Once you have the api-mock-service mock server running, you can upload contracts using:
curl -H "Content-Type: application/yaml" --data-binary @fixtures/get_customer.yaml \
http://localhost:8000/_scenarios
curl -H "Content-Type: application/yaml" --data-binary @fixtures/save_customer.yaml \
http://localhost:8000/_scenarios
You can start your service before invoking generated tests, e.g. we will use sample-openapi for the testing purpose and then invoke the generated tests using:
curl -X POST http://localhost:8000/_contracts/customers -d \
'{"base_url": "http://localhost:8080", "execution_times": 5, "verbose": true}'
Above command will execute all tests for customers group and it will invoke each REST API 5 times. After executing the APIs, it will generate result as follows:
{
"results": {
"get-customer_0": {
"email": "anna.intra@amicum.edu",
"id": "fa7a06cd-1bf1-442e-b761-d1d074d24373"
},
"get-customer_1": {
"email": "aaron.sequi@laetus.gov",
"id": "c5128ac0-865c-4d91-bb0a-23940ac8a7cb"
},
"get-customer_2": {
"email": "edward.infligi@evellere.com",
"id": "a485739f-01d4-442e-9ddc-c2656ba48c63"
},
"get-customer_3": {
"email": "gary.volebant@istae.com",
"id": "ef0eacd0-75cc-484f-b9a4-7aebfe51d199"
},
"get-customer_4": {
"email": "alexis.dicant@displiceo.net",
"id": "da65b914-c34e-453b-8ee9-7f0df598ac13"
},
"save-customer_0": {
"email": "anna.intra@amicum.edu",
"id": "fa7a06cd-1bf1-442e-b761-d1d074d24373"
},
"save-customer_1": {
"email": "aaron.sequi@laetus.gov",
"id": "c5128ac0-865c-4d91-bb0a-23940ac8a7cb"
},
"save-customer_2": {
"email": "edward.infligi@evellere.com",
"id": "a485739f-01d4-442e-9ddc-c2656ba48c63"
},
"save-customer_3": {
"email": "gary.volebant@istae.com",
"id": "ef0eacd0-75cc-484f-b9a4-7aebfe51d199"
},
"save-customer_4": {
"email": "alexis.dicant@displiceo.net",
"id": "da65b914-c34e-453b-8ee9-7f0df598ac13"
}
},
"errors": {},
"metrics": {
"getcustomer_counts": 5,
"getcustomer_duration_seconds": 0.006,
"savecustomer_counts": 5,
"savecustomer_duration_seconds": 0.006
},
"succeeded": 10,
"failed": 0
}
Though, generated tests are executed against real services, it’s recommended that the service implementation use test doubles or mock services for any dependent services as contract testing is not meant to replace component or end-to-end tests that provide better support for integration testing.
Recording Consumer/Producer interactions for Generating Stub Requests and Responses
The contract testing does not always depend on API specifications such as Open API and swagger and instead you can record interactions between consumers and producers using api-mock-service tool.
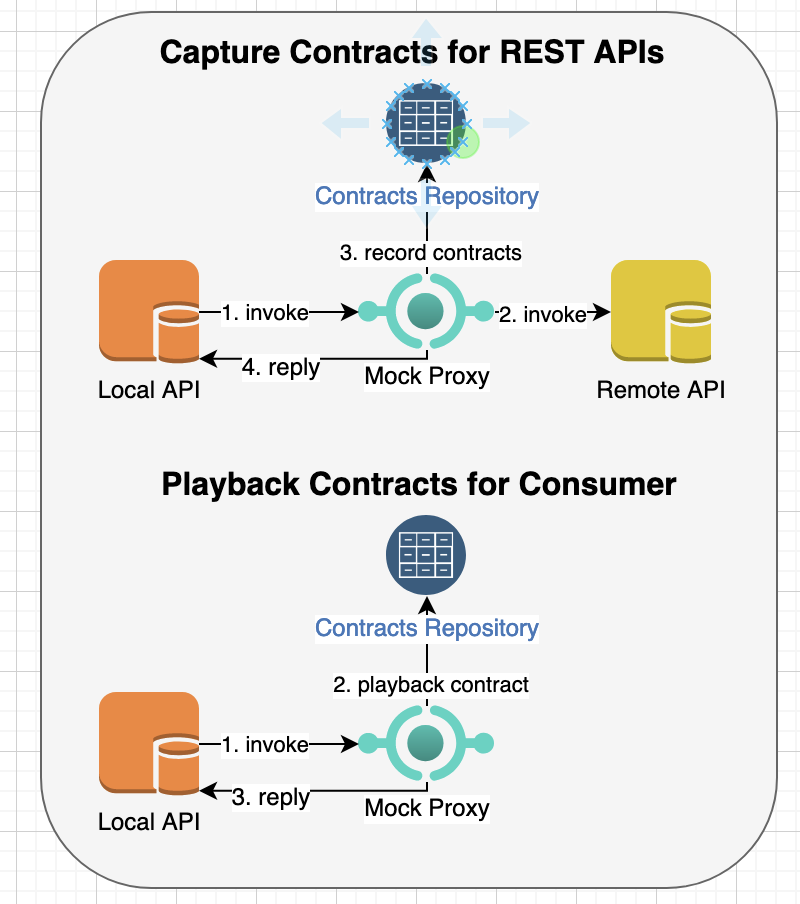
For example, if you have an existing REST API or a legacy service such as above sample API, you can record an interaction as follows:
export http_proxy="http://localhost:9000"
export https_proxy="http://localhost:9000"
curl -X POST -H "Content-Type: application/json" http://localhost:8080/customers -d \
'{"address":{"city":"rwjJS","countryCode":"US","id":"4a788c96-e532-4a97-9b8b-bcb298636bc1","streetAddress":"Cura diu me, miserere me?","zipCode":"24121"},"creditCard":{"balance":{"amount":57012,"currency":"USD"},"cardNumber":"5566-2222-8282","customerId":"tgzwgThaiZqc5eDwbKk23nwjZqkap7","expiration":"70/6666","id":"d966aafa-c28b-4078-9e87-f7e9d76dd848","type":"VISA"},"email":"andrew.recorder@ipsas.net","firstName":"quendam","id":"071396bb-f8db-489d-a8f7-bbcce952ecef","lastName":"formaeque","phone":"1-345-6666-0618"}'
This will invoke the remote REST API, record contract interactions and then return server response:
{
"id": "95d655e1-405e-4087-8a7d-56791eaf51cc",
"firstName": "quendam",
"lastName": "formaeque",
"email": "andrew.recorder@ipsas.net",
"phone": "1-345-6666-0618",
"creditCard": {
"id": "d966aafa-c28b-4078-9e87-f7e9d76dd848",
"customerId": "tgzwgThaiZqc5eDwbKk23nwjZqkap7",
"type": "VISA",
"cardNumber": "5566-2222-8282",
"expiration": "70/6666",
"balance": {
"amount": 57012,
"currency": "USD"
}
},
"address": {
"id": "4a788c96-e532-4a97-9b8b-bcb298636bc1",
"streetAddress": "Cura diu me, miserere me?",
"city": "rwjJS",
"zipCode": "24121",
"countryCode": "US"
}
}
The recorded contract can be used to generate the stub response, e.g. following configuration defines the recorded contract:
method POST
name recorded-customers-200-55240a69747cac85a881a3ab1841b09c2c66d6a9a9ae41c99665177d3e3b5bb7
path /customers
description recorded at 2023-01-02 03 18 11.80293 +0000 UTC for http //localhost 8080/customers
order0
group customers
predicate""
request
match_query_params
match_headers
Content-Type application/json
match_contents'{"address.city":"(__string__\\w+)","address.countryCode":"(__string__\\w+)","address.id":"(.+)","address.streetAddress":"(__string__\\w+)","address.zipCode":"(__string__\\d{5,5})","creditCard.balance.amount":"(__number__[+-]?\\d{1,10})","creditCard.balance.currency":"(__string__\\w+)","creditCard.cardNumber":"(__string__\\d{4,4}[-]\\d{4,4}[-]\\d{4,4})","creditCard.customerId":"(.+)","creditCard.expiration":"(.+)","creditCard.id":"(.+)","creditCard.type":"(__string__\\w+)","email":"(__string__\\w+.?\\w+@\\w+.?\\w+)","firstName":"(__string__\\w+)","id":"(.+)","lastName":"(__string__\\w+)","phone":"(__string__\\d{1,1}[-]\\d{3,3}[-]\\d{4,4}[-]\\d{4,4})"}'
path_params
query_params
headers
Accept'*/*'
Content-Length"522"
Content-Type application/json
User-Agent curl/7.65.2
contents'{"address":{"city":"rwjJS","countryCode":"US","id":"4a788c96-e532-4a97-9b8b-bcb298636bc1","streetAddress":"Cura diu me, miserere me?","zipCode":"24121"},"creditCard":{"balance":{"amount":57012,"currency":"USD"},"cardNumber":"5566-2222-8282","customerId":"tgzwgThaiZqc5eDwbKk23nwjZqkap7","expiration":"70/6666","id":"d966aafa-c28b-4078-9e87-f7e9d76dd848","type":"VISA"},"email":"andrew.recorder@ipsas.net","firstName":"quendam","id":"071396bb-f8db-489d-a8f7-bbcce952ecef","lastName":"formaeque","phone":"1-345-6666-0618"}'
example_contents""
response
headers
Content-Type
application/json
Date
Mon, 02 Jan 2023 03:18:11 GMT
contents'{"id":"95d655e1-405e-4087-8a7d-56791eaf51cc","firstName":"quendam","lastName":"formaeque","email":"andrew.recorder@ipsas.net","phone":"1-345-6666-0618","creditCard":{"id":"d966aafa-c28b-4078-9e87-f7e9d76dd848","customerId":"tgzwgThaiZqc5eDwbKk23nwjZqkap7","type":"VISA","cardNumber":"5566-2222-8282","expiration":"70/6666","balance":{"amount":57012.00,"currency":"USD"}},"address":{"id":"4a788c96-e532-4a97-9b8b-bcb298636bc1","streetAddress":"Cura diu me, miserere me?","city":"rwjJS","zipCode":"24121","countryCode":"US"}}'
contents_file""
example_contents""
status_code200
match_headers
match_contents'{"address.city":"(__string__\\w+)","address.countryCode":"(__string__\\w+)","address.id":"(.+)","address.streetAddress":"(__string__\\w+)","address.zipCode":"(__string__\\d{5,5})","creditCard.balance.amount":"(__number__[+-]?\\d{1,10})","creditCard.balance.currency":"(__string__\\w+)","creditCard.cardNumber":"(__string__\\d{4,4}[-]\\d{4,4}[-]\\d{4,4})","creditCard.customerId":"(.+)","creditCard.expiration":"(.+)","creditCard.id":"(.+)","creditCard.type":"(__string__\\w+)","email":"(__string__\\w+.?\\w+@\\w+.?\\w+)","firstName":"(__string__\\w+)","id":"(.+)","lastName":"(__string__\\w+)","phone":"(__string__\\d{1,1}[-]\\d{3,3}[-]\\d{4,4}[-]\\d{4,4})"}'
pipe_properties
assertions
wait_before_reply 0s
You can then invoke consumer-driven contracts to generate stub response or invoke generated tests to test against producer implementation as described in earlier section. Another benefit of capturing test contracts using recorded session is that it can accurately capture all URLs, parameters and headers for both requests and responses so that contract testing can precisely validate against existing behavior.
Summary
Though, unit-testing, component testing and end-to-end testing are a common testing strategies that are used by most organizations but they don’t provide adequate support to validate API specifications and interactions between consumers/clients and producers/providers of the APIs. The contract testing ensures that consumers and producers will not deviate from the specifications and can be used to validate changes for backward compatibility when APIs are evolved. This also decouples consumers and producers if the API is still in development as both parties can write code against the agreed contracts and test them independently. A service owner can generate producer contracts using tools such as api-mock-service based on Open API specification or user-defined constraints. The consumers can provide their consumer-driven contracts to the service providers to ensure that the API changes don’t break any consumers. These contracts can be stored in a source code repository or on a registry service so that contract testing can easily access them and execute them as part of the build and deployment pipelines. The api-mock-service tool greatly assists in adding contract testing to your software development lifecycle and is freely available from https://github.com/bhatti/api-mock-service.